Understanding Data CF: A Comprehensive Guide
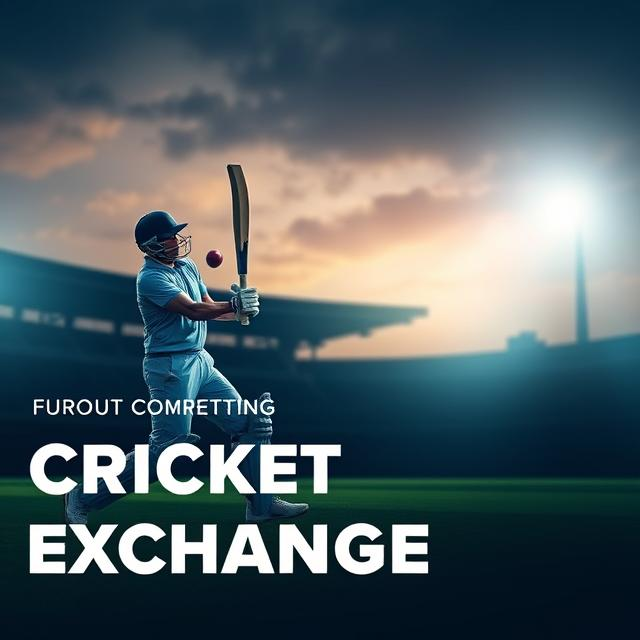
Unveiling the Power of Collaborative Filtering (CF) in Data
In today’s data-driven world, understanding and harnessing the power of different data analysis techniques is crucial for informed decision-making. One such technique, Collaborative Filtering (CF), is rapidly gaining traction for its ability to uncover hidden patterns and insights within vast datasets. This article delves into the core concepts of data CF, exploring its applications and limitations. Ready to unlock the secrets hidden within your data?
What is Collaborative Filtering (CF)?
Collaborative filtering is a popular recommendation system technique that works by analyzing the preferences of numerous users. By identifying users with similar tastes and preferences, it predicts what a user might like based on what others with similar tastes have liked. Imagine recommending movies based on what your friends have enjoyed! That’s the essence of CF. Data CF builds on this principle by applying it to broader datasets, enabling it to make more precise and accurate recommendations.
How Does Data CF Work?
Data CF algorithms typically operate by identifying patterns in user-item interactions. This could be explicit feedback, like ratings, or implicit feedback, such as purchase history or clicks. The core of the method is to group users with similar preferences and then leverage these groups to predict future user behavior or identify latent patterns in the data.
Crucially, these algorithms analyze a vast amount of data, allowing for more sophisticated predictions and tailored recommendations based on individual preferences. This approach often leads to recommendations more relevant to the user, thereby enhancing the user experience.
Applications of Data CF
The applications of data CF are diverse and far-reaching, impacting various industries.
- E-commerce: Recommending products to customers based on their browsing history and purchase patterns.
- Online Video Streaming: Suggesting videos and movies based on viewing history and preferences.
- Social Media Platforms: Recommending friends, content, and even ads based on connections and interests.
- Finance: Identifying potential risk factors and providing personalized investment recommendations based on market trends and individual investor behavior.
Limitations of Data CF
While data CF is a powerful technique, it does have limitations. For example, the accuracy of recommendations can suffer from the “cold start” problem – predicting preferences for new users or items with limited interaction data. Furthermore, data CF can sometimes fall prey to biases present within the training data, leading to less accurate or unfair recommendations for some user groups.
Conclusion
Understanding data CF’s core principles and applications is crucial in today’s digital landscape. By harnessing the power of this technique, businesses can leverage user preferences to provide enhanced customer experiences, leading to greater engagement and profitability. Data CF is continuously evolving and will likely play an increasingly important role in shaping the future of data analysis.